More than half the patients affected by Hepatitis C for interferon don’t respond to treatment with interferon. Scientists use mathematical modeling to understand why and gain insight into how the treatment can be made more successful.
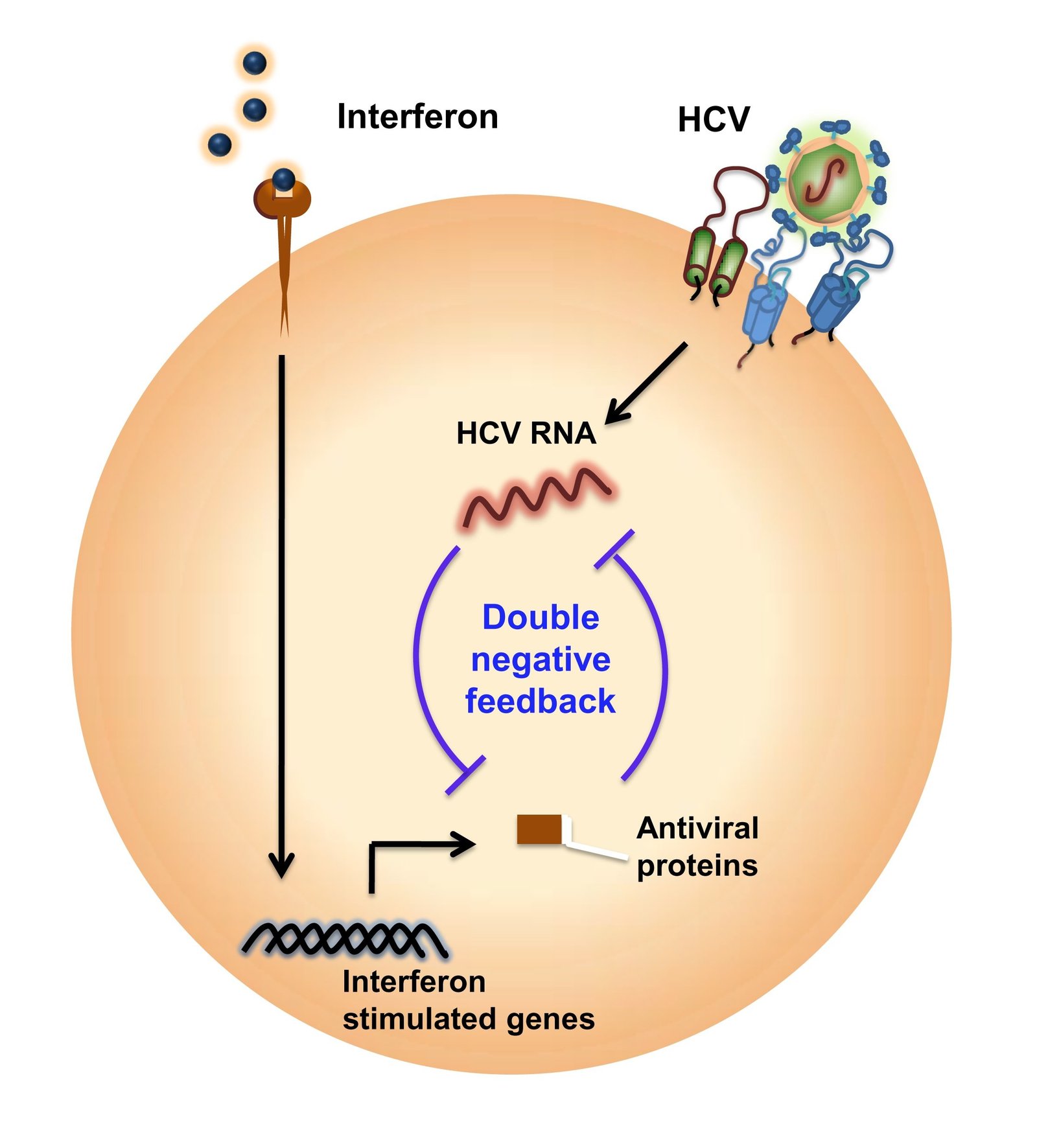
Hepatitis C, an infectious disease that affects the liver, is caused by the Hepatitis C virus (HCV). About 200 million people are infected with the virus worldwide and over 10 million of them are from India. Currently, the most common treatment for the infection is a combination of interferon and ribavirin. However, only about 50% of patients respond to this treatment. Why the treatment fails was not well understood, until now.
“We wanted to find out why the treatment of HCV with interferon doesn’t work as well as it should,” said Narendra Dixit, Associate Professor in the Department of Chemical Engineering at the Indian Institute of Science (IISc), Bangalore. Dixit and his colleagues used a cell-signaling model that qualitatively explains how HCV interacts with the interferon network and why it evades treatment in such a large number of patients.
Interferons are naturally occurring proteins that are made by our cells in the presence of pathogens, including viruses and tumor cells. The release of interferon kicks-off a complex series of signaling events that results in the expression of hundreds of genes called interferon-stimulated genes. These genes activate the defense mechanisms of the immune system against viruses.
The response of HCV to interferon, or the lack thereof, presents an intriguing paradox. Previous studies by others have measured the extent to which naturally produced interferon was acting — before treatment was begun. What they found, rather surprisingly, was that the genes that are triggered by interferon were expressed to a larger extent in patients who did not respond to interferon treatment.
The attempts to explain this paradox have, so far, unraveled specific interactions of the virus with the interferon-signaling network. “These findings have not brought us any closer to distinguishing non-responders to treatment or given us strategies to improve treatment. Our thought was that the answer probably didn’t lie in these individual interactions. The difference between patients who respond to treatment and those who don’t must lie at the systemic level,” said Dixit.
To test their hypothesis they constructed a mathematical model of the interferon-signaling network and its interaction with HCV to capture the battle between the virus and the network that tries to overcome it. Interferon-stimulated genes suppress HCV, while HCV affects interferon activity, resulting in a double-negative feedback. “The underlying physics is not very difficult. It is the scale which makes the model complex and difficult to understand, because there are a large number of molecules involved and they are interacting in some complex ways,” explained Dixit. Their next step was to “solve the model” and extract properties exhibited by the network.
What they found was that HCV introduces bistability in the system. This result, published in their recent paper in Nature Communications is crucial — it provides a theoretical basis for understanding the poor response of HCV to interferon treatment in some patients. A system that is bistable can stabilize in one of two states. In this case, the first stable steady state is the one where interferon wins and HCV infection is cleared. The second steady state is one where the virus persists. In this state, no amount of perturbation with additional treatment affects the virus and it continues to thrive.
Which of these states a cell is in can vary, even within one individual, owing to intrinsic variations in the cells’ machinery. While some cells are in the state where the virus persists, others are in the state where interferon wins and the virus is cleared. A third population of cells can swing either way — these cells are easily susceptible, and exposure to the virus or interferon drives them to the corresponding steady state. How a patient responds or how he deals with the infection depends on which of these cell populations is predominant. “There is no obvious way for us to predict that,” said Dixit.
The model helps to resolve the paradox of why the treatment fails in some patients even when they have high interferon-stimulated gene expression. They have more cells in the state where the virus is able to overcome the interferon response and persist.
More recently, direct-acting antiviral agents or DAAs have been approved for treating HCV, in addition to interferon treatment. DAAs act on viral proteins to block proliferation. These have proved successful in patients who could not be treated with interferon. By identifying the steady states of the network when different doses of DAAs are administered, the researchers show that the DAAs transform a bistable cell into one that only admits a state where interferon works to clear the HCV infection.
“It was nice that this property showed up in this model. It explains, at least qualitatively, what interferon is doing. In addition, this model is not just restricted to treatments that involve interferon. It also gives us basic insight into how the virus interacts with the system and how that can be tweaked to allow the underlying interferon response to reach its maximum potential,” summarized Dixit.