Researchers from Jadavpur University have developed classification-based machine-learning models for toxicity assessment of plastic packaging associated chemicals. These models can efficiently predict the toxicity of chemicals thereby providing a valuable tool for researchers as well as regulatory bodies, overcoming health concerns.
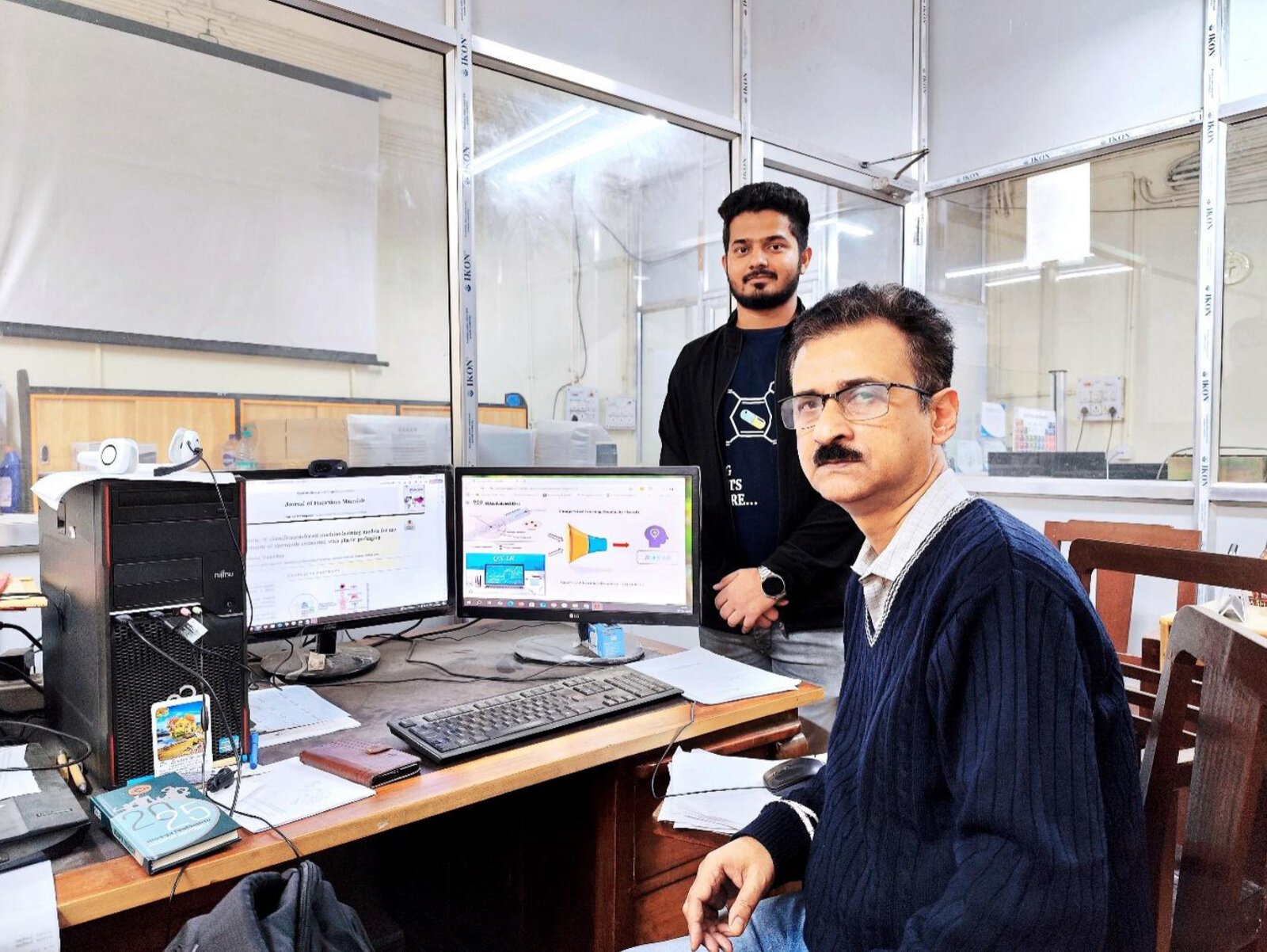
The global plastic production and rising demand of plastic packaging which often comprises complex polymers, additives, and residual chemicals poses a significant risk to consumers due to the accumulation of toxic chemicals into the consumer products like food, cosmetics as well as environment, throughout the plastic’s lifecycle. Many of these chemicals are hazardous, impacting human health and wildlife. With the aim of reducing these risks, a team led by Kunal Roy from DTC lab, Jadavpur University have developed machine learning models that can efficiently and accurately predict toxicity of chemicals. Their study published in the Journal of Hazardous Materials, provided a machine-learning framework enabling rapid screening of chemicals. “The study addresses a critical gap in chemical safety evaluation by providing interpretable insights into chemical toxicity”, Roy highlighted.
The study analysed an extensive dataset consisting of over 6,700 chemical compounds focusing on seven key toxicity endpoints: neurotoxicity, hepatotoxicity, dermatotoxicity, carcinogenicity, reproductive toxicity, skin sensitisation, and toxic pneumonitis that may occur from exposure to chemicals in plastic packaging.
Data imbalance (uneven distribution in the number of samples across classes i.e., toxic and non toxic outcomes in this study) was observed in training (subset to train model) and test (subset to assess model’s performance) dataset which can lead to biased models resulting in reduced sensitivity and misleading conclusions. Thus, the dataset was balanced with four over-sampling and three under-sampling approaches generating seven balanced datasets suitable for a robust model. “The innovative use of data balancing techniques effectively addresses class imbalance, improving model reliability across multiple toxicity endpoints”, said Nirav Limbasiya, a board certified toxicologist and senior consultant at ToxMinds with more than a decade of experience in the field of toxicology and who is not associated with this study. The models were based on four classification approaches with their individual parameters, optimised by means of five-fold cross-validation. In addition, SHAP (SHapley Additive exPlanations) analysis was used to enhance the model’s interpretability. It also indicated key chemical descriptors for predicting toxicity associated with each toxicity endpoint. “The transparency established through machine learning allowed an understanding of chemical behaviour, as well as structural determinants causing toxicity”, Roy added.
Furthermore, the Sum of Ranking Differences (SRD) method was used to evaluate and systematically rank each machine learning model, thereby providing an objective means to identify the optimal model.
Roy explained, “This unbiased ranking technique ensured that the most capable models from each toxicity endpoint were selected, most models exhibiting 80% and above accuracy.”
Overall, the developed model provides a scalable and a rapid method with high accuracy. “The integration of SHAP analysis enhances interpretability by identifying key molecular descriptors influencing toxicity, thus, making the predictions more transparent, while the SRD method provides an objective model selection approach”, Limbasiya added. Unlike traditional toxicity assessments, which are labour-intensive and time-consuming, developed machine-learning framework enables rapid screening of chemicals which makes it a constructive tool for researchers as well as industries. The study also paves way for safer design and usage of plastic materials by efficiently interpreting chemical toxicity. Further expansion of the model’s applicability by integrating a wide array of chemical datasets will enhance the robustness of tools for toxicity screening.