Our brains are quite proficient at recognizing jumbled words and reading them correctly. Researchers from the Indian Institute of Science, Bengaluru, studied this fascinating phenomenon and came up with a computational model that uses artificial neurons to simulate the way the brain processes jumbled words.
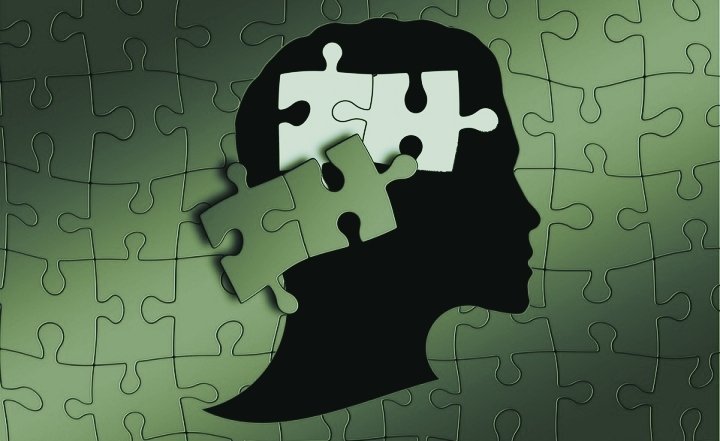
How does our brain read jumbled words correctly? Scientists led by SP Arun and K V S Hari from the Centre for Neuroscience, Indian Institute of Science (IISc), Bengaluru, have developed a computational model that sheds light on this. According to this model, when we see a string of letters, our brain uses the letter shapes to form an image of the word and compares it with the closest visually similar word stored in our brain.
Reading words is a complex process in which our brain decodes the letters and symbols in the word (also called the orthographic code) to derive meaning. Earlier research has shown that our brain processes jumbled words at various levels — visual, phonological and linguistic.
At the visual level, it is easy to read a jumbled word correctly when the first and the last letters are retained and the other letters are jumbled or replaced with letters of similar shapes. Yet, some arrangements are easier to read than others. For instance, ‘UNIEVRSITY’ is easier to read than ‘UTISERVNIY.’ We can also read words when numbers of similar shape replace letters, e.g. “7EX7__WI7H__NUM83R5.”
At a linguistic level, it is easier to recognize words that we encounter more frequently or have frequently-used letters. At the phonological level, it is easier to recognize similar sounding words, e.g. tar/car, pun/fun etc. However, how these factors contribute individually or collectively to recognize words remains unclear.
“We show that our ability to read jumbled words comes from simple rules in the visual system, whereby the response to a string of letters is a weighted sum of its individual letters,” Aakash Agarwal, first author of the paper, says.
The team asked fluent English speakers aged 22 – 27 years to search for the odd letter out within a group of letters (distractors) displayed on the screen. The researchers found that the more similar were the shapes of the odd letter and the distractors, the more time the subjects took to accurately spot it. The team could thus calculate an index of how similar or different English letters were to each other, based on the time taken by the subjects to spot the odd letter in this experiment.
Using this information, the team proceeded to design computational units (artificial neurons) that were mathematically tuned to gauge how similar or different letters were to each other, thereby mimicking the neurons in the brain. Using these artificial neurons, the team then predicted how much time human subjects would take to identify odd two-letter combinations hidden within an array of two-letter distractors and found that the predictions matched the experimental findings.
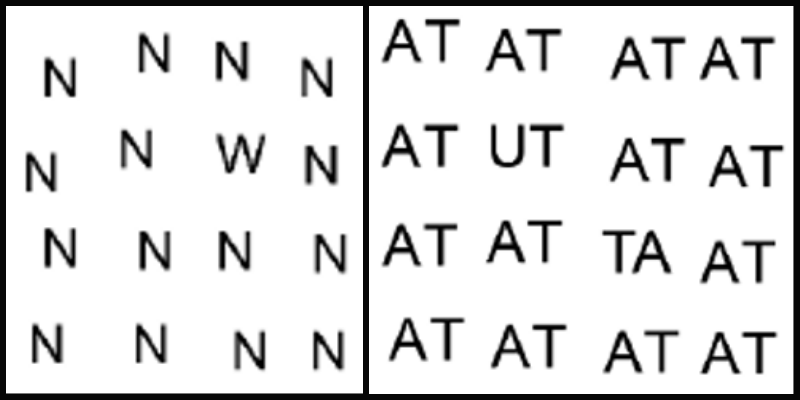
The researchers then tested the responses of the artificial neurons to four, five and six-letter words, studying how difficult or easy it was for these neurons to distinguish actual words from jumbled words. The more similar the shape of the jumbled word was to the correct word, the more difficult it was to identify it as jumbled. It was also more difficult to spot jumbled words when the first and the last letters were kept the same. For example, it was easier to spot EPNCIL as a jumbled word than PENICL.
The artificial neurons processed these words by adding up the responses to individual letters contained within the word. They could also predict the time that the human brain would take to correctly identify a non-jumbled word from within a group of jumbled words. This was confirmed by experimental findings.
Finally, the researchers used functional MRI to capture brain images of volunteers performing a word recognition task to see which region of the brain got activated during the process. They found that observing a word activates the lateral occipital region – the part of the brain that processes visual information. Following this, the brain compares it with similar-looking words, which is probably stored in the visual word form area (VWFA).
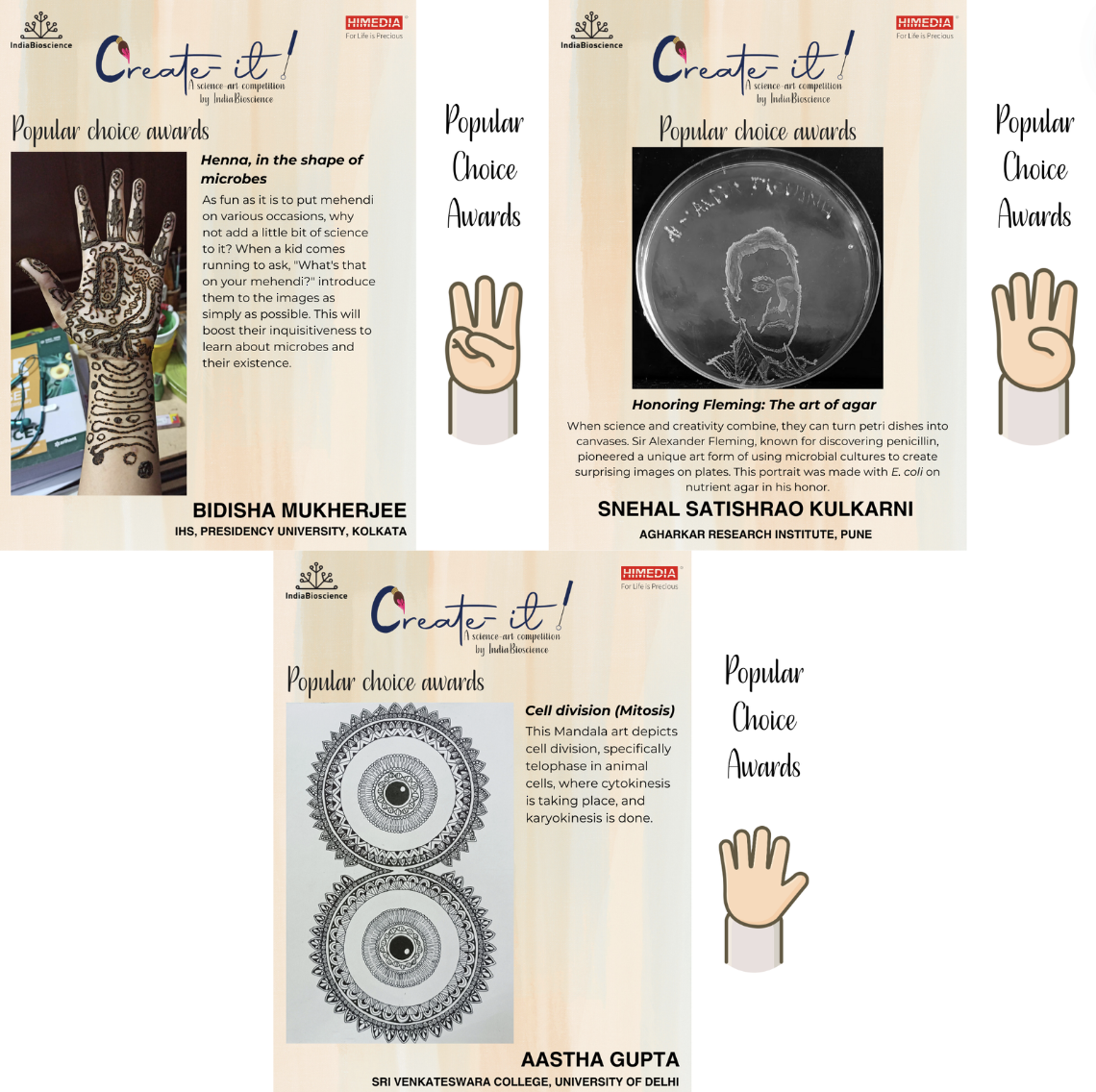
“We hope that our model would address the shortcoming of existing models that aim to crack the orthographic code and compel researchers to reconsider the contribution of vision in orthographic processing,” Agarwal says.
Arpan Banerjee, Additional Professor, National Brain Research Centre (NBRC), who was not involved in the study, says that one unique aspect of this study is how computational modelling has been used to explain the neural data.
The findings could help in developing efficient text recognition algorithms and also enable better diagnosis of reading disorders like dyslexia. “It would be interesting to see how this model can work for more complex languages like Hindi and Urdu,” Banerjee says. He also wonders if the properties of the artificial neurons would change after learning two languages.
The team intends to explore the role of visual processing in predicting reading fluency in children. “While deficits in phonological processing are the main cause for dyslexia, a subset of children has deficits at the visual level. We aim to identify this subset through visual experiments and develop training regimes to help improve their reading fluency in our next follow-up study,” Agarwal says.